Affective Computing
MIT research director, Dr. Rosalind Picard, introduced the concept of affective computing technology in 1997 through the release of her book “Affective Computing”. Her breakthrough work sparked a wave of research on the emotional interactions between humans and machines, leading to what we now call Emotion Artificial Intelligence (AI).
While Dr. Picard’s work on affective computing started over 25 years ago, we have only witnessed a fraction of its full capabilities.
How does it work?
Affective computing continues to grow as technology advances and learns to interact with humans in more natural and intuitive ways. At its core, this important technology combines psychology, computer science, and engineering to develop systems and devices that recognize, interpret, and respond to human emotions.
Basically, Affective Computing combines psychology, computer science and engineering to develop systems and devices that recognize, interpret and respond to human emotions.
As Techslang.com explains, affective computing works by “…collecting user data through physical sensors, such as video cameras and microphones, and analyzing such information based on previous experiences and data sets.” The technology uses emotion recognition to recognize different emotions, such as joy or sadness, and can also generate appropriate emotional responses. For example, an affective computing system might be able to recognize that a person is feeling sad and respond by playing a soothing song or displaying a comforting message.
Affective Computing and Emotion AI
Affective computing and Emotion AI are essentially the same thing. With Affective Computing we usually refer to the engineering and computational side of Emotional Artificial Intelligence while with Emotion AI we refer to the psychological and socio-economic side. Both have borrowed and automated cognitive neuroscience tools making them faster and easier to use, optimizing time and resources.
These technologies use facial expression recognition software to record action units: measurements of facial muscles to classify emotional expressions.
Action units were created by Paul Ekman and Wally Friesen through their work on the Facial Action Coding System (FACS). Ekman and Friesen identified a total of 40 action units and hundreds of combinations. Action units help with categorizing emotions.
Why does affective computing matter?
Dr. Picard originally conceived affective computing as she recognized that “there had to be something like emotional reasoning for there to be any form of true machine intelligence.” This new technology changes the game of artificial intelligence and its potential contribution to society as it helps improve the human-computer interaction experience.
Many potential use cases exist, including these three examples:
- Education: Teachers can understand and respond to their students’ emotional states in real-time, which improves the learning experience through personalized feedback and support
- Healthcare: Healthcare workers can monitor and respond to their patients’ emotional states, which can help improve patient care by providing real-time feedback and support based on their emotional state
- Customer service: Customers can enjoy an improved experience through personalized support and assistance based on their emotional state; For example, recognizing when a customer is upset using additional tools such as sentiment analysis
Emotion AI
We’ve put together this “Glossary of Emotion AI” to help you understand everything you need to know to take advantage of this growing AI tool and all of its relevant techniques.
SEE HOW IT WORKS
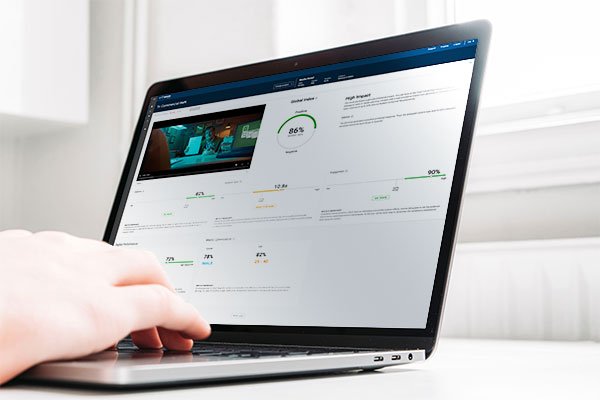