A Glossary of Emotion AI: Understanding the Key Terms and Concepts
Emotion Artificial Intelligence or Emotion AI – this relatively new technology is gaining traction in several use cases from conducting market research to powering empathetic robots. But what exactly is Emotion AI, what are the key terms and concepts involved, and how will it make a positive impact for society?
Table of Contents
What is Emotion AI?
Emotion AI uses affective computing to create a set of systems that can recognize, interpret, and in some cases respond to human emotions. Affective computing is a type of artificial intelligence that deals with the analysis of human affective states. These tools today use different human signals to encode affective states:
- Prosodic: the “music of language” that helps us understanding the meaning by the way words are said (e.g., ending a sentence in a high-pitch tone might sound like a question)
- Body posture: the way our body forms and moves as we react to certain situations (e.g., we might slouch our shoulders when we’re feeling insecure)
- Facial expressions: the way our facial muscles form and move as we react to certain stimuli (e.g., the corners of our lips might curve up into a smile when we hear a baby laughing)
The origin of Emotion AI
The field of Emotion AI is relatively new, but the study of emotions has long existed, thanks to a few pioneers in the psychology field. Here are a few of the many.
Charles Darwin
In 1872, Charles Darwin published a theory arguing (Scientific American) “… all humans, and even other animals, show emotion through remarkably similar behaviors.” Darwin conducted a small experiment to test human recognition, which is still used today in modern psychology experiments.
Paul Ekman
Paul Ekman has long contributed to the field of emotions, such as creating the ‘Wheel of Emotions’, which we will cover later. In 1978, Ekman, along with fellow psychologist and researcher Wally Friesen, developed the Facial Action Coding System (FACS), a comprehensive system of facial measurement that anyone, even scientists, could use to understand facial expressions. Ekman and Friesen created a catalog of action units, the movement of a facial muscle or muscle groups that configure the expression of an emotion, which power the FACS. Ekman discovered seven primary emotions easily recognizable by facial expressions: joy, sadness, fear, disgust, surprise, anger, and contempt (experts debate if this is a distinct emotion).
Ekman later wrote a paper (1997), “The Universal Facial Expressions of Emotions”, where he found: “…strong evidence of universality of some facial expressions of emotion as well as why expressions may appear differently across cultures.” Ekman’s work provides a foundation for many emotion-based tools as his focus was on identifying primary emotions easily recognizable by facial expressions and thus measurable.
Robert Plutchik
In 1980, college professor Robert Plutchik introduced the “Wheel of Emotions” to help others understand the different types of possible emotions and levels of intensity. Plutchik’s research found over 30,000 emotions but was narrowed down to eight primary emotions in polar opposite pairs (Toolshero.com):
- Joy vs. Sadness
- Trust vs. Disgust
- Fear vs. Anger
- Anticipation vs. Surprise
In addition to the “Wheel of Emotions”, Plutchik wrote “A Psychoevolutionary Theory of Emotions”, which details the evolutionary origins of emotions, helping to provide a framework to unify several perspectives. Plutchik’s theory provides several important characteristics, including these few (Plutchik via ScienceDirect.com):
- A broad evolutionary foundation for conceptualizing the domain of emotion as seen in animals and humans
- A structural model for interrelations among emotions
- Theoretical and empirical relations among a number of derivative domains including personality traits, diagnoses, and ego defenses
- A theoretical rationale for the construction of tests and scales for the measurement of key dimensions within these various domains
>>RELEVANT BLOG POST: HOW EMOTION COULD DRIVE YOUR CAMPAIGNS TO SUCCEED
Donald E. Brown
In 1990, Dr. Brown wrote a book called Human Universals focused on challenging a common anthropological topic: whether human beings across different demographics share universal behavioral traits. Dr. Brown conducted six case studies, with one focused on facial expressions of emotion. Dr. Brown found that human beings shared a set of basic expressions, following several studies featuring subjects from different cultures.
Emotiva
In 2017, Emotiva launched with a purpose to develop computer vision and machine learning algorithms to analyze people’s emotional responses in real-time, measuring facial micro-expressions through a standard webcam, to collect data useful for understanding human behaviors.
Emotiva’s work in affective computing has pushed the use cases of Emotion AI in new ways from conducting market research to creating an emotional brain for social robotics and measuring the emotional engagement of art through a new measurement technique called the Stendhal Index.
The key terms and concepts
Emotion AI involves several key terms and concepts:
- Emotions
- Emotion recognition
- Sentiment analysis
- Emotion generation
- Affective computing
- Human-computer interaction
- Facial expression recognition
- Natural language processing
- Facial Coding
Understanding emotions
We all know what emotions feel like, but scientifically, emotions are “a complex blend of actions, expressions, and internal changes to the body that occur in response to the meaning we make of our environment,” as written by Carolyn MacCann, Ph.D.
Read more about how understanding emotions.
Emotion recognition
Even human beings can have a tough time recognizing certain emotions. Emotion recognition refers to the use of technology, specifically machine learning algorithms, to identify a person’s emotions by measuring facial expressions, body movements, or vocal cues. Emotion AI tools measure emotion recognition with four key elements: action units, face geometry, appearance, and relations between each.
Read more about the emotion recognition definition.
Sentiment analysis
Some people express their emotions better in writing. Sentiment analysis looks at written text to determine the emotional tone. Several companies use this for social media monitoring or gauging customer feedback.
Read more about how sentiment analysis.
Emotion generation
As defined by IGI-Global, emotion generation is the “…process of generating emotions based on perceived external stimuli” to replicate human-like behavior as much as possible. It is creating an artificial feeling through natural language processing, facial animation, or other techniques.
Read more about how emotion generation.
Affective computing
As mentioned earlier, Emotion AI, also known as affective computing, involves the systems and devices to detect, analyze, and respond to human emotions in a natural way. Affective computing combines several disciplines: psychology, engineering, and computer science.
Read more about how affective computing.
Human-computer interaction
Human-computer interaction focuses on the design, evaluation, and implementation of interactive computer systems. Emotion AI has the potential to greatly enhance human-computer interaction by making interactions with computers more natural and intuitive, and by allowing computers to respond to a user’s emotional state in a more meaningful way.
Read more about how human-computer interaction.
Facial expression recognition
In the context of emotion AI, facial expression recognition uses machine learning algorithms, which are trained on large datasets of labeled images, and standard webcams that detect subtle changes in the facial muscles, to identify and categorize facial expressions.
Read more about how facial expression recognition.
Natural language processing
IBM defines natural language processing as building “…machines that understand and respond to text or voice data—and respond with text or speech of their own—in much the same way humans do.” Emotion AI uses natural language processing to process and interpret text or speech data.
Read more about how natural language processing.
Facial Coding
The FACS system is used by attributing a combination of codes corresponding to certain facial micro-movements (Action Units) made by the subject. Furthermore, it is possible to identify an intensity of the movement and the combination of these movements can lead to a subsequent decoding or a “translation” of the code into a predominantly emotional meaning.
How it works
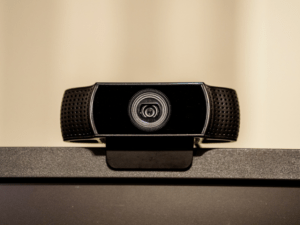
Now that we have a good understanding of the origins and key terms of Emotion AI, let’s dive into how Emotion AI works. Emotion AI involves several concepts to identify and classify emotions as demonstrated above, but computer vision is what is used to digitally capture the emotions. In the context of Emotion AI, computer vision uses standard webcams to identify and measure micro-facial expressions and encode emotional responses through action unit classification.
What are action units
Action units represent the facial muscles or combination of facial muscles that form the expression of an emotion, based on Ekman’s work on the FACS. The FACS includes 40 action units and hundreds of action unit combinations, providing a rich library to support Emotion AI tracking.
Tracking action units – step by step
The process for tracking action units using Emotiva’s technology starts like this:
- A standard webcam captures people’s reactions (facial expressions, head movements to certain stimuli, without any further configuration.
- Emotion AI software processes the image to extract the first features, face geometry, and appearance.
- The action unit classifier tool identifies each individual muscle activation and intensity.At the end of the analysis process, the algorithm shows the expressions of emotions identified according to four levels of emotional states (primary, secondary, compound, and state) by correlating the active action units.
Use cases
Emotion AI is leveraged by several industries for its ability to provide an empathetic layer to research and analysis. Emotion AI becomes even more powerful when combined with attention measurement, which identifies attention level and attention span. This enhanced approach enables empowered decision making.
Here are a few Emotion AI use cases.
Market research
Marketing teams constantly search for key performance metrics that indicate success. For most teams, market research starts in the early stages of a campaign. Take for example NeN, an Italian energy company. NeN had made a six-figure investment in creating new commercials for a new TV campaign. They needed to make sure they used the right commercials that would provide the most level of engagement. NeN leveraged Emotion AI to analyze attention and emotional engagement levels on each video to ultimately select the most effective one.
Robotics
The robotics field continues to evolve, with Emotion AI introducing a new way to test human-to-robot interactions. A new humanoid robot by the name of Abel made headlines for its empathetic capabilities. Using Emotion AI technology, Abel learns how to improve its empathetic capabilities and human interactions by reading and extracting emotions and reacting appropriately.
Art
Emotion AI has the potential to disrupt the art industry. Some might say that art equals emotions and Emotion AI can capture which works of art have the most emotional impact. A cryptoart startup called REASONEDArt used a new Emotion AI metric called the Stendhal Index for one of its recent exhibitions to measure emotional responses to each art piece.
Automotive
Emotion AI might be leveraged by the automotive industry in a slightly different way from the previous three use cases. The use case here is more about safety, measuring attention levels of those operating dangerous equipment and cannot afford to be distracted.
Healthcare
Similar to the robotics use case, Emotion AI can have significant impact in the healthcare field as it relates to helping patients manage neurodevelopmental and neurodegenerative disorders. There’s also potential in helping communicate with non verbal patients, such as those with autism or Alzheimer patients.
Human resources
Another potential use case involves the use of Emotion AI to measure attention and emotional engagement levels during important company trainings. Human resources managers can get a good understanding when certain parts of a training are confusing based on employee reactions.
Why is Emotion AI important
Emotion AI can provide different benefits depending on the use case, as mentioned above. But one of the biggest drivers for investing in Emotion AI is that attention is limited. Numerous studies show that today’s population is easily distracted, taking only three seconds to capture someone’s attention before they move on to something else. The attention economy makes it imperative to understand what engages your audience.
Another reason why Emotion AI is important is that it allows you to measure what otherwise might be intangible. Yes, you can capture someone’s thoughts through a survey. But as Ekman and many others have pointed out, the one thing you cannot deny and exist across cultures are emotions. What’s more, Emotion AI opens the door not only for smarter decision making, but also predicting future results through predictive analytics.
How to get started
Getting started with Emotion AI is easy, especially with a cloud-based tool such as EmPower. It takes a few minutes to set up your analysis, about 2-3 days to get your initial results, and your dashboard will instantly show attention and emotional engagement levels.
SEE HOW IT WORKS
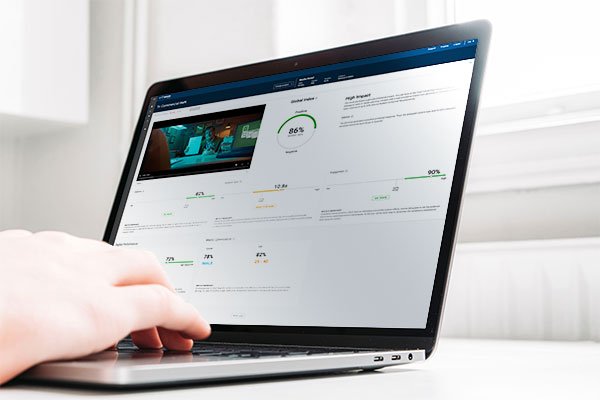