Facial Expression Recognition
Micro facial expression recognition may not be quite what you think. It involves more than just scanning a face, at least in regards to how it relates to the Emotion AI.
With Emotion AI, the recognition of facial microexpressions is based on facial muscle activations to understand the emotional impact of the person when interacting with something.
How does it work?
Facial expression recognition uses machine learning algorithms that are trained on large datasets of labeled facial expressions, which can include images, videos, or even 3D scans of faces. The algorithms learn to recognize patterns in the data to help with recognizing emotion (check out our emotion recognition definition article for more information.) For example, a smiling face might be associated with happiness, while a furrowed brow might indicate anger or frustration.
Furthermore, it is necessary to specify that the algorithms trained to classify the action units have a higher level of accuracy than those that use neural networks trained, therefore, to decode the expressions and detect the emotion.
While machine learning algorithms are a powerful tool for recognizing facial microexpressions, they can have some limitations. For example, factors like lighting and even the angle of a face could affect the results.
How does facial expression recognition support Emotion AI?
Facial expression recognition supports Emotion AI by collecting the right data needed for processing. These software analyze facial muscle movements to determine the emotional response based on facial expressions, thus helping to assess a person’s emotional impact in a given context.
Action units help determine the expression of emotions through their correlations and can have huge benefits in enhancing human-machine interaction experiences.
Why does facial expression recognition matter?
Facial expression recognition provides several benefits and has a wide range of applications, from mental health to market research.
There is much potential to help in several different situations, such as diagnosing and treating mental health conditions. For example, a mental health professional could monitor a patient’s facial expressions and emotions during a therapy session. This could help the therapist identify patterns of emotion that might be associated with certain mental health conditions, such as depression or anxiety.
Facial expression recognition can also be used to support market research. For example, with Emotion AI, marketers can better understand which types of media will have the most potential to influence their audience based on their emotional reactions, thus driving their campaigns to succeed.
How to learn more
Overall, facial expression recognition has the potential to revolutionize a wide range of fields. By using machine learning algorithms and specialized sensors, it is possible to accurately identify emotions based on facial expressions. This technology has the potential to improve mental health care, improve marketing campaign efficiency, and even help improve our understanding of human emotions.
If you would like to learn more about how many factors contribute to Emotion AI, check out this Glossary of Emotion AI. We are also available to help answer any questions. Reach out to the team today.
SEE HOW IT WORKS
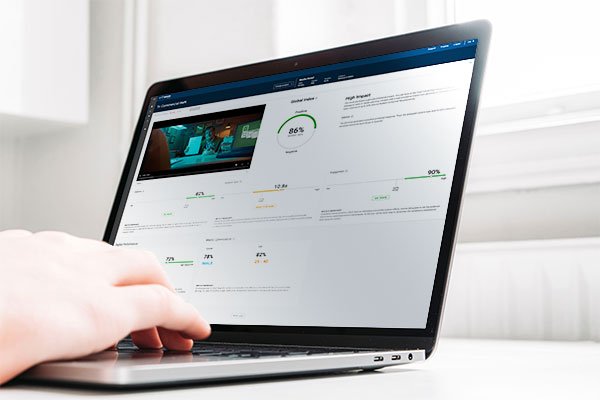